The environmental costs of AI
Exploring the environmental impacts of AI in language services and the steps we can take as a profession to address them.
The increasing use of AI technologies such as neural machine translation (NMT) and large language models (LLMs) in language services is already a hot topic, with translators and interpreters raising questions about ethics, transparency and quality etc. Many ITI members are also concerned about the significant environmental costs arising from the training and use of AI. We therefore felt that it is important to inform members about this aspect of the AI debate and asked Dr Matthew Riemland to share his expertise.
The hidden environmental costs of AI
The material infrastructures required to train and operate AI models are expansive. Most models are trained and hosted in remote data centres via cloud computing services, where thousands of processing chips linked together on server racks are designed to handle computationally intensive AI workloads. Activists around the world have protested the construction of data centres due to their environmental harms, which are exacerbated by exploding AI demand. But while data centres represent key sites in AI infrastructures, AI’s ecological impacts extend far beyond these locations, implicating complex global supply chains with many actors. These supply chains’ complexity, scale, and general lack of transparency make it exceedingly difficult to attain accurate figures regarding precise damages. However, it’s still important to have a general overview of the severity and multi-faceted nature of AI’s environmental harms:
The price of AI's energy demands
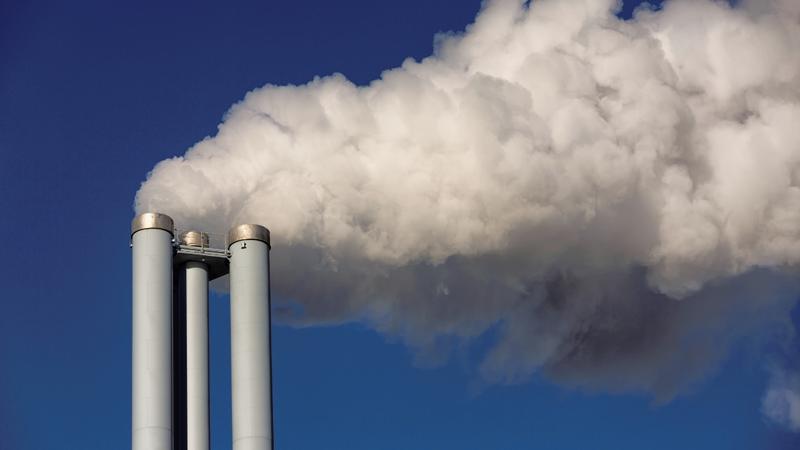
Language AI generates massive carbon emissions. Data centres require staggering amounts of energy in order to process AI workloads. And while energy mixes vary greatly by region, energy is often sourced via fossil fuels, generating carbon emissions. Rather alarmingly, a recent study anticipates that the air pollution caused by data centres’ increased operations may lead to major upticks in public health issues and even premature deaths in surrounding areas.
There are two primary emissions-generating phases of AI workloads: training and inference. During the training phase, AI systems cycle through massive amounts of training data in order to construct the highly sophisticated mathematical model that it will later use to process inputs and generate outputs. The inference phase is when users use a model for its intended purposes, such as entering a source-language segment into an NMT system to generate a corresponding target-language segment.
The training phase is particularly energy-intensive and can generate substantial carbon emissions. Researchers Dr Sasha Luccioni and Dr Alex Hernandez Garcia examined the training conditions of nearly 95 AI models and estimated their aggregate training emissions to be 253 tons of CO2eq – roughly the same emissions as 100 flights from London to San Francisco. But while a single inference or use of an AI model consumes very little energy and thus generates a tiny fraction of the carbon emitted during training, the model’s repeated, ongoing use may cumulatively consume much more energy – particularly for highly popular, publicly available AI models. Inference may therefore account for far greater emissions than training at prominent tech companies like Google, for example. (Again, emissions are highly dependent on data centres’ locations.)
And although these factors are highly important in assessing AI models’ carbon impacts, they only reflect operational emissions. We must also consider AI infrastructures’ embodied emissions, which refer to the carbon emissions generated across all activities in a product’s or service’s supply chain, including materials extraction, production, transport, and manufacturing. Crucially, hardware innovations that bring reductions in AI models’ operational emissions often lead to comparable or even greater increases in embodied emissions. This trend has led some researchers to predict that AI’s embodied emissions will surpass its operational emissions in the future. Regardless, the growing AI sector’s exorbitant energy demands are in many cases prolonging regions’ reliance on fossil fuels, creating more emissions.
In response to the emissions generated by AI, many developers and tech companies turn to carbon offsetting, although this practice is highly controversial. It is perhaps encouraging that there are a handful of digital tools designed to help developers estimate the carbon emitted by their AI models. However, these tools exclude embodied emissions – not least due to the lack of transparency across supply chains – and there is very little agreement between them.
AI's strain on water resources
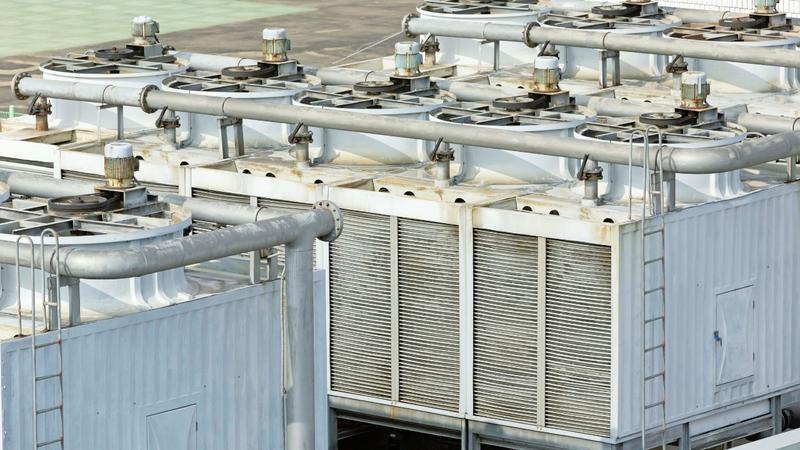
Language AI consumes substantial amounts of water, often placing an additional burden on water-stressed communities. The computational intensity of AI workloads puts data centre hardware at risk of overheating, requiring a reliable method of keeping machinery cool in order to operate. The most common cooling method in data centres is the use of cooling towers, which take in water and release heat through evaporation. This direct form of water consumption is complemented by AI’s indirect water consumption, referring to water consumed in order to produce energy. (Indirect water consumption varies greatly according to the energy source, but is generally substantial.)
As with carbon emissions, AI’s water consumption largely depends on the location of the data centres in which it operates. Some researchers have estimated that GPT-3’s training phase – executed in Microsoft’s US-based data centres – directly consumed approximately 700,000 litres of clean freshwater for cooling purposes, and indirectly consumed some 2.8 million litres of water from electricity generation. The same research team estimated that ChatGPT (based on GPT-3) consumes the equivalent of a 500 ml bottle of water in processing a user interaction involving 20-50 prompts and outputs. Given the popularity of this particular AI model, it is not hard to imagine how the service’s massive user base could collectively consume staggering quantities of water. In drought-stricken regions like the southwestern United States, data centres are consuming more and more water due to rapidly growing AI demand, competing with local communities and agriculture for a substantial portion of an already-depleted water supply. Data centres’ water consumption has also caused concern in London.
To make matters worse, water efficiency is often at odds with carbon efficiency: data centres in sunny regions with abundant solar energy – great for reducing operational carbon emissions– need more water to cool machinery due to the hotter climate. This predicament illustrates the complex entanglement of AI’s ecological impacts.
Mining for AI: the human and ecological toll of rare minerals
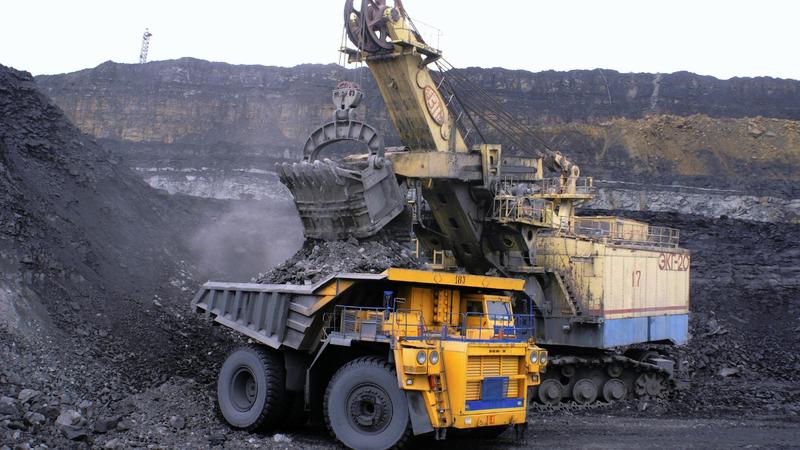
AI infrastructures require many types of metals and rare minerals whose extraction is linked to mining pollution and severe human rights abuses. Key components of the hardware needed to operate AI models consist of metals and rare minerals such as cobalt, copper, gallium, lithium, and tungsten. Cobalt, for instance, is overwhelmingly sourced from the Democratic Republic of the Congo, where the cobalt mining industry is rife with forced labour, life-threatening conditions and other human rights violations. A good portion of the world’s lithium supply comes from the Lithium Triangle in Argentina, Bolivia and Chile, where lithium mining has caused serious pollution in local ecosystems and exacerbated water scarcity. The widespread adoption of AI services and subsequent expansion of AI infrastructures have undoubtedly increased demand for these critical resources, thus magnifying the negative effects of their extraction. Even in carbon-efficient data centres relying primarily on renewable energy, the various metals and rare minerals necessary for their hardware components are directly linked to social and environmental harms in other parts of the world.
And crucially, making individual models more resource-efficient may lead to rebound effects that boost the overall consumption of AI across society, ironically causing AI’s aggregate environmental costs to increase
So what can we do? Steps for the translation and interpreting profession
Admittedly, the picture painted here is quite grim, and there is a lot of work to be done in order to reckon with AI’s environmental harms. Different actors in the translation and interpreting industries have different roles to play in tackling this issue.
Language service providers (LSPs) and developers working with NMT and LLMs can be more proactive in providing estimates of the environmental impacts of their AI models and publish information on the various contributing factors to the greatest extent possible. Digital tools can help them make adjustments to software and operations to improve models’ carbon efficiency. CodeCarbon – compatible with major cloud providers like Amazon Web Services, Microsoft Azure, and Google Cloud Platform — allows developers to approximate their model’s carbon footprint while suggesting ways to lower it. Online calculators like ML CO2 Impact and Green Algorithms as well as python packages like energy-usage and cumulator similarly offer fairly straightforward means of estimating carbon emissions from AI. Google Cloud Platform and Microsoft Azure also offer their own in-built calculators. But as alluded to above these tools are generally inconsistent and incomplete, relying on simplistic representations of complex ecological processes and social responsibility. LSPs can publicise the precise conditions of their model training/operations and use these limited tools to provide tentative estimates of their carbon impacts while advocating for broader sectoral change. Moreover, it is crucial that LSPs reflect on whether the benefits of the particular AI models they develop and deploy are worth the environmental costs they incur.
Translators can encourage clients and LSPs working with AI to disclose the factors that influence AI’s carbon impact, perhaps emphasising the importance of data centre location in determining emissions. They can also locate data centres in the local area and take action to improve transparency and raise awareness of their environmental impacts. As with LSPs, translators can reflect on their own use of AI tools and their environmental consequences. But there are significant pressures on translators to work with AI tools in order to adjust to an increasingly tech-reliant industry, and it would be neither fair nor realistic to implore them to abstain from these technologies altogether. Ultimately, confronting AI’s environmental harms will require broader social change targeting not only carbon emissions but also the many other ecological consequences of these sophisticated digital infrastructures. ITI remains convinced that the translation and interpretation industries can serve as leaders in this area.
Transparency is just the first step
The lack of transparency in the material aspects of AI’s supply chain is a major issue, and the problem may be even more severe than we realise. Still, proper disclosure is not guaranteed to bring about a solution to AI’s environmental harms; we’ll need to go beyond making individual models as resource-efficient as possible and ask what uses of language AI justify their environmental costs.
If you would like to get involved with ITI’s efforts to promote better environmental practices in the translation and interpreting industries, please contact [email protected].